
Deezer’s magic Flow is now available in Moods
As Lead Data Scientist at Deezer, Benjamin Chapus is in charge of personalizing the platform using advanced statistics and machine learning. Thanks to the data collected, each Deezer user finds a tailor-made home page that follows their listening habits and preferences, and a Flow that offers custom recommendations.
The Deezer Flow
Since 2014, Deezer has offered Flow, a feature that generates a personalized soundtrack to your day. The algorithm is based on the user’s musical preferences, to create a mix of favorite tracks and tailored discoveries. “Listening to too many unknown tracks can be annoying. So we apply classic radio rules with a regular occurrence of familiar songs to reassure the ear. This is the ideal moment to make a musical discovery.”
For Benjamin Chapus, Flow is some sort of magic playlist. “On Twitter, we sometimes read that Flow has magically played a song that’s been bugging someone. It’s nice to hear but quite logical, because if the person has been thinking of that song, it means they’ve been exposed to it, so the likelihood of the song making it into their Flow is pretty high. Sometimes, there are also some miraculous linkages, hyper sharp: had we tried to do it on purpose, we wouldn’t have succeeded. And sometimes it’s not just by chance, sometimes Flow can read the user’s thoughts, and that’s quite magical.”
Flow Moods: a variation
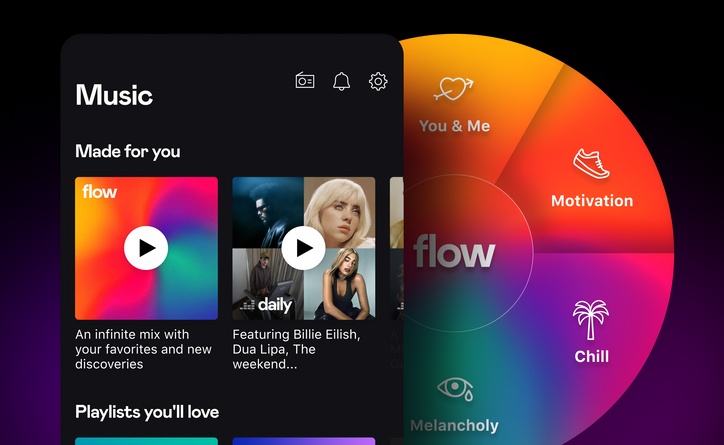
At the beginning, Flow was supposed to remain very simple, with a single play button. The promise was strong: Deezer was committed to finding what people wanted to listen to. However, even if Flow is magical, it is impossible to guess the user’s mood. Since music is often associated with an activity, Deezer decided to diversify the formula.
With Flow Moods, launching in October 2021, the user chooses the mood they’re in, allowing Flow to explore different genres, with smoother transitions. “Until now, only playlists offered music matching a context. But it’s not very complicated to educate an algorithm that way, since music classification techniques are based on the audio signal.”
Millions of data to analyze
Each week, the French streaming platform receives hundreds of millions of songs, which Benjamin Chapus’ team must manage. To qualify the music, the Data Scientists can rely on provided data, on the behavioral data of a song or on the audio signal.
First, the metadata, provided by distributors like IDOL, or labels directly. “This data indicates the name of the artist, the genres associated with an album, and the artist’s nationality for instance.” Then, behavioral data, based on user actions. “Take a song put in several rap playlists, and listened to by people who are used to listening to rap music, that’s accumulated trustworthy indices.” Finally, the audio signal. “There are a lot of open-source algorithms that will break the back of the work and analyze the audio signal, to extract a number of high-level descriptors, such as BPM, pitch or musical genre.” Then, this data must be cross-referenced to offer a tailored recommendation.
A large music map
To consolidate these researches, Benjamin Chapus’ team sends bots out on the web to inspect public data, on Wikipedia, Discogs, and even music blogs like Pitchfork. “Because these reviews are written by a human, it allows us to find influences or associated sub-genres, which broadens the aura of an album.”
Among the tens of thousands of albums received each day, not all of them are eligible to the algorithm. That would be too much data to process, so it needs some sorting out. “We receive a lot of garbage, like reissues, sometimes royalty-free songs, or even sounds: beach sounds, bird songs, or vacuum cleaner noise. You have to be able to understand what’s important, hence the interest in checking if an album finds an echo in the music news.”
All of these elements help position the piece on a big music map. “We cannot generalize a style for an artist, who can have a diverse musical signature along his career. It’s all these subtleties that we try to capture, to create relevant recommendations.”
A minimalist design
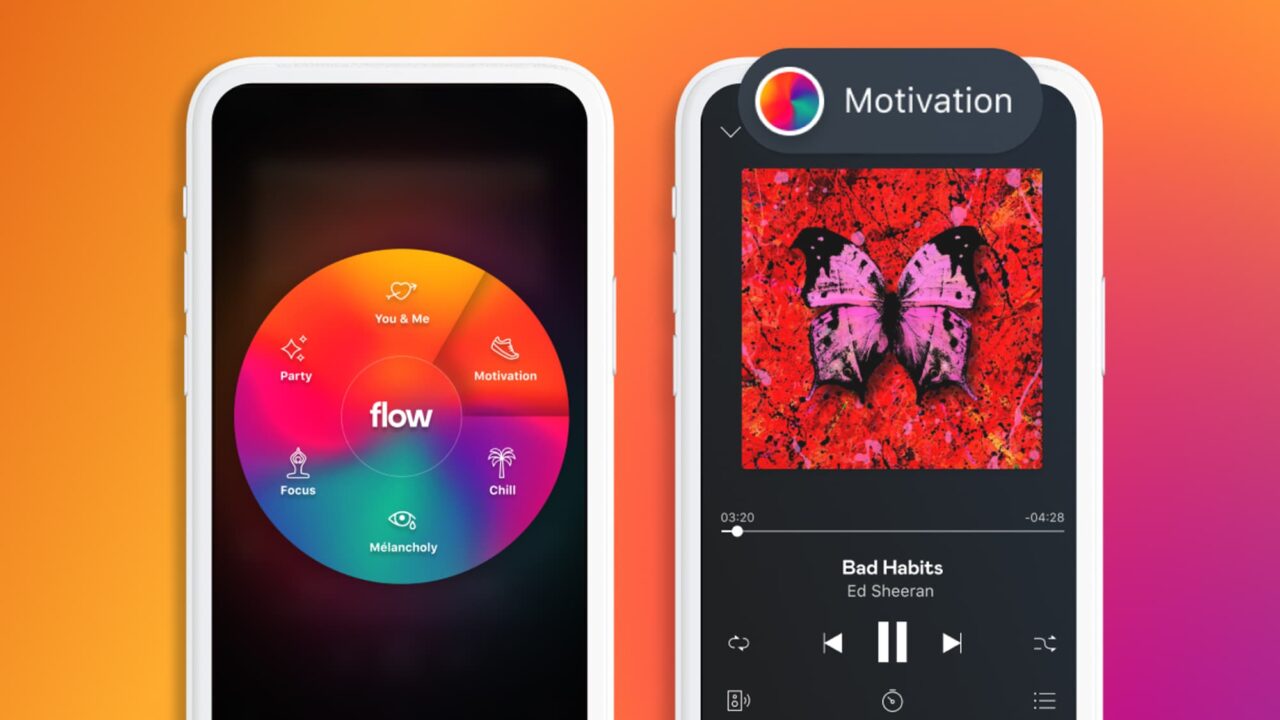
The more we listen to music, the more the recommendation is relevant. Because with each action on the platform, we indicate our tastes to the algorithm. On Flow, there are only a few buttons: play, forward, rewind, and especially like and ban. These last two buttons are the main signals to inform the Flow.
On the user’s profile, the Data Science team analyzes all the listenings, based on this large map of music, with large continents of music style, which sometimes come together. “All these elements help us define three or four musical interests, and Flow will juggle between these profile barycenters. If someone tends to skip a lot of the songs played, Flow will change musical style.”
When someone bans an artist or a song, Flow creates a blacklist while trying to figure out if the problem comes from the artist or the style. “We test, kind of like a battleship, aiming right next to the target. Little by little, the algorithm learns, according to the actions taken, and Flow tries to converge as quickly as possible, to what people want to listen to.”
Full-scale tests
The advantage of working on a platform like Deezer is that the Data Science team will be able to conduct large-scale tests to study user behavior. But also, to sharpen the algorithms thanks to the in-house playlists. “We have a very large editorial team that develops a lot of thematic playlists, which makes the selection work very easy.”
Because in order to create a suitable “motivational” playlist, you have to present the algorithm with “motivational” songs from all musical styles. This data collection can be very tedious. “And having this eclectic, comprehensive and varied selection is a real asset. Once that curation is done, the algorithm part is pretty easy to roll out.”
Follow Deezer
Official Website / Facebook / Twitter / Instagram / YouTube / TikTok / Snapchat /
The community